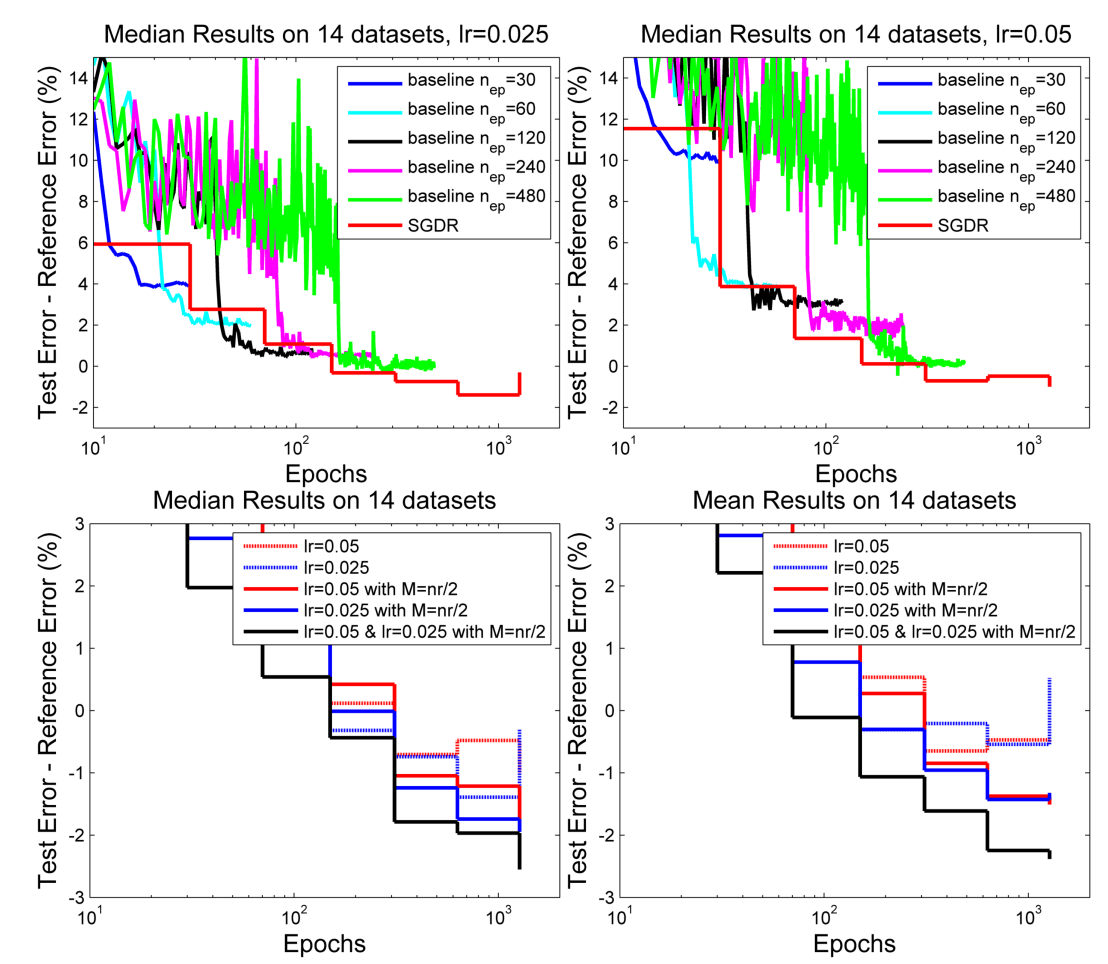
SGDR: Stochastic Gradient Descent with Warm Restarts
Date : 2016-05-03
Abstract
Restart techniques are common in gradient-free optimization to deal with multimodal functions. Partial warm restarts are also gaining popularity in gradient-based optimization to improve the rate of convergence in accelerated gradient schemes to deal with ill-conditioned functions. In this paper, we propose a simple warm restart technique for stochastic gradient descent to improve its anytime performance when training deep neural networks. We empirically study its performance on the CIFAR-10 and CIFAR-100 datasets, where we demonstrate new state-of-the-art results at 3.14% and 16.21%, respectively. We also demonstrate its advantages on a dataset of EEG recordings and on a downsampled version of the ImageNet dataset.
Research paper below links to GitHub repo
Recently on :
Artificial Intelligence
Research
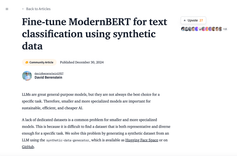
WEB - 2024-12-30
Fine-tune ModernBERT for text classification using synthetic data
David Berenstein explains how to finetune a ModernBERT model for text classification on a synthetic dataset generated from argi...
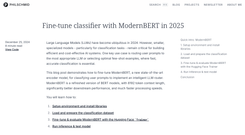
WEB - 2024-12-25
Fine-tune classifier with ModernBERT in 2025
In this blog post Philipp Schmid explains how to fine-tune ModernBERT, a refreshed version of BERT models, with 8192 token cont...
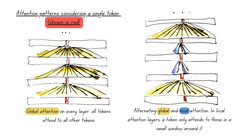
WEB - 2024-12-18
MordernBERT, finally a replacement for BERT
6 years after the release of BERT, answer.ai introduce ModernBERT, bringing modern model optimizations to encoder-only models a...
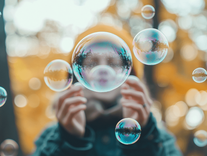
PITTI - 2024-09-19
A bubble in AI?
Bubble or true technological revolution? While the path forward isn't without obstacles, the value being created by AI extends ...
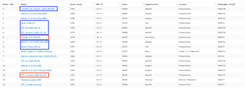
PITTI - 2024-09-08
Artificial Intelligence : what everyone can agree on
Artificial Intelligence is a divisive subject that sparks numerous debates about both its potential and its limitations. Howeve...