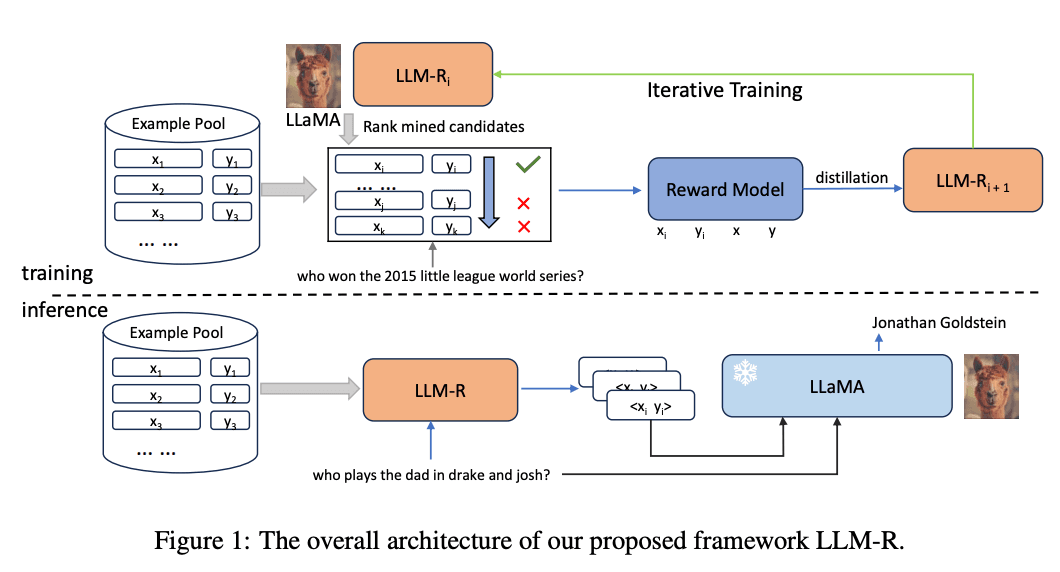
Abstract
Large language models (LLMs) have demonstrated their ability to learn in-context, allowing them to perform various tasks based on a few input-output examples. However, the effectiveness of in-context learning is heavily reliant on the quality of the selected examples. In this paper, we propose a novel framework to iteratively train dense retrievers that can identify high-quality in-context examples for LLMs. Our framework initially trains a reward model based on LLM feedback to evaluate the quality of candidate examples, followed by knowledge distillation to train a bi-encoder based dense retriever. Our experiments on a suite of 30 tasks demonstrate that our framework significantly enhances in-context learning performance. Furthermore, we show the generalization ability of our framework to unseen tasks during training. An in-depth analysis reveals that our model improves performance by retrieving examples with similar patterns, and the gains are consistent across LLMs of varying sizes.
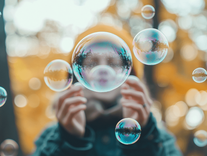
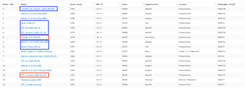
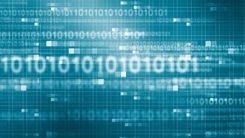
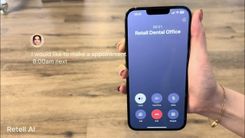
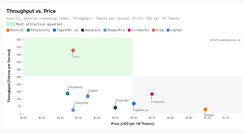