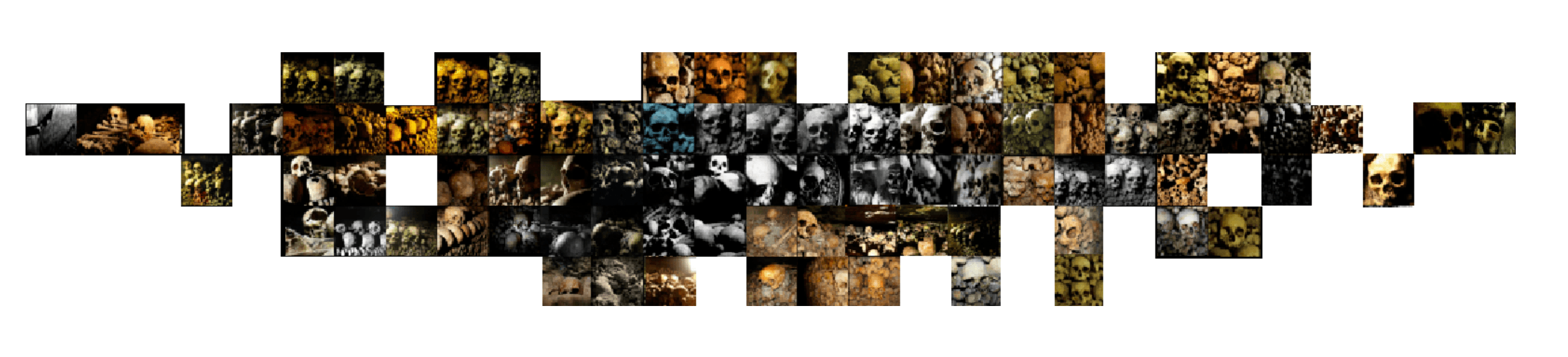
Abstract
By looking at the politics of classification within machine learning systems, this article demonstrates why the automated interpretation of images is an inherently social and political project. We begin by asking what work images do in computer vision systems, and what is meant by the claim that computers can “recognize” an image? Next, we look at the method for introducing images into computer systems and look at how taxonomies order the foundational concepts that will determine how a system interprets the world. Then we turn to the question of labeling: how humans tell computers which words will relate to a given image. What is at stake in the way AI systems use these labels to classify humans, including by race, gender, emotions, ability, sexuality, and personality? Finally, we turn to the purposes that computer vision is meant to serve in our society—the judgments, choices, and consequences of providing computers with these capacities. Methodologically, we call this an archeology of datasets: studying the material layers of training images and labels, cataloguing the principles and values by which taxonomies are constructed, and analyzing how these taxonomies create the parameters of intelligibility for an AI system. By doing this, we can critically engage with the underlying politics and values of a system, and analyze which normative patterns of life are assumed, supported, and reproduced.
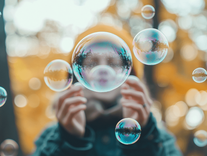
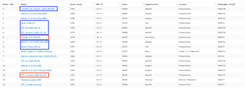
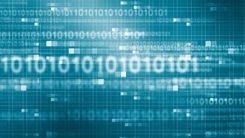
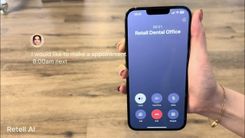
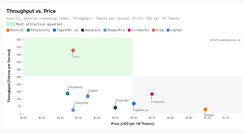