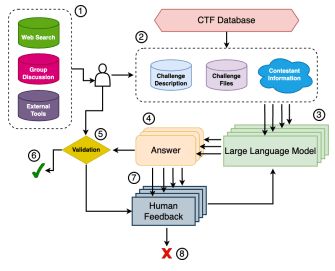
Abstract
Capture The Flag (CTF) challenges are puzzles related to computer security scenarios. With the advent of large language models (LLMs), more and more CTF participants are using LLMs to understand and solve the challenges. However, so far no work has evaluated the effectiveness of LLMs in solving CTF challenges with a fully automated workflow. We develop two CTF-solving workflows, human-in-the-loop (HITL) and fully-automated, to examine the LLMs' ability to solve a selected set of CTF challenges, prompted with information about the question. We collect human contestants' results on the same set of questions, and find that LLMs achieve higher success rate than an average human participant. This work provides a comprehensive evaluation of the capability of LLMs in solving real world CTF challenges, from real competition to fully automated workflow. Our results provide references for applying LLMs in cybersecurity education and pave the way for systematic evaluation of offensive cybersecurity capabilities in LLMs.
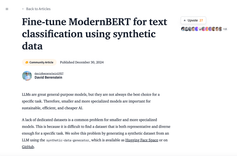
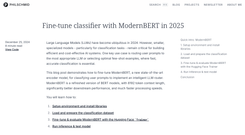
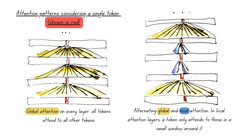
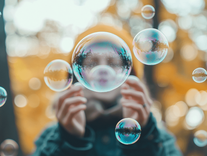
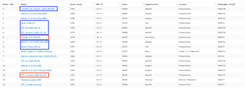