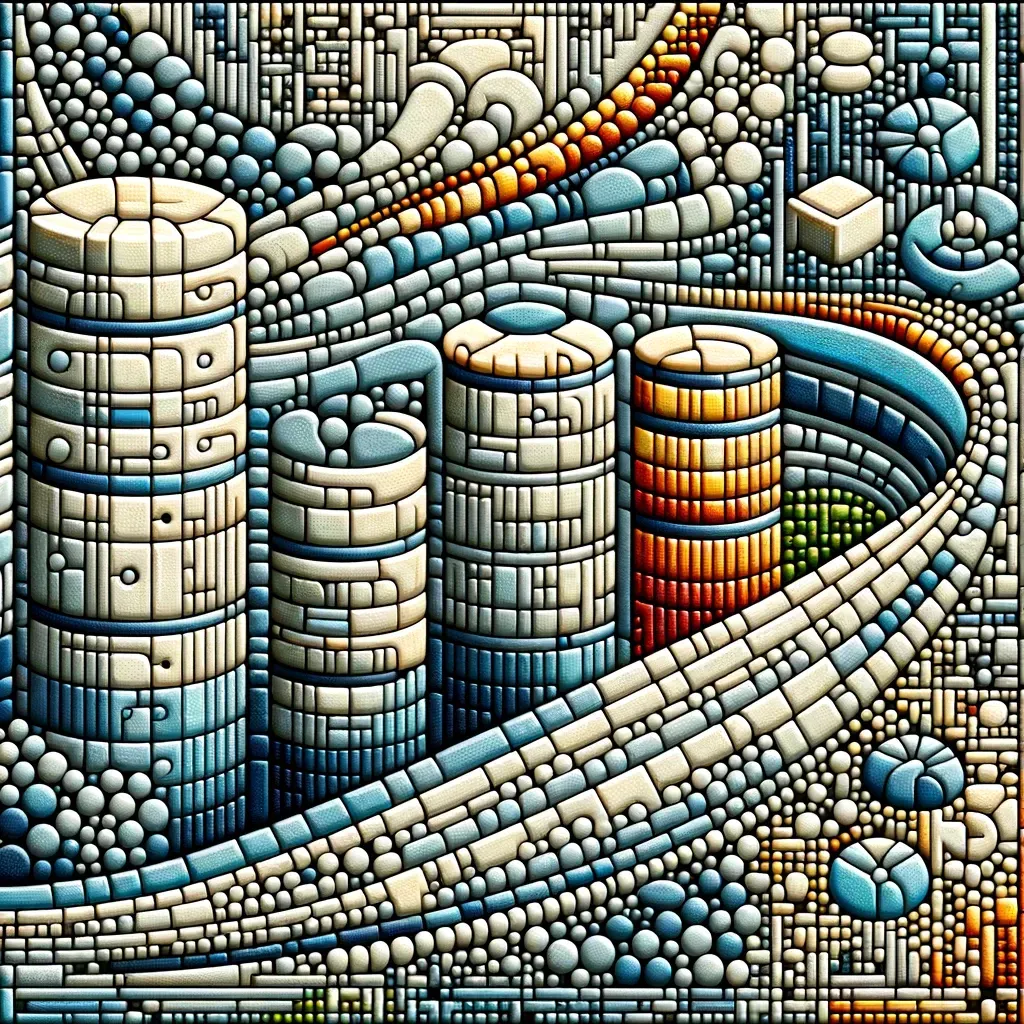
Description
In his article, Panda Smith challenges the common perception of vector databases as providing expanded memory for language models, stating that they are ultimately just a type of search. Instead, he emphasizes the importance of building a good search engine for creating effective RAG tools using language model-powered documentation experiences. Shipilov shares his experience with using traditional vector search and finding it insufficient in delivering accurate results. He highlights the benefits of combining keyword and embedding search to achieve better performance than pure fulltext search. Furthermore, he introduces the concept of using language models to structure queries for optimal information retrieval. Additionally, he explains how recent advancements in AI have made it easier to build cutting-edge search engines, significantly reducing the required time and effort. Shipilov concludes by underlining the importance of evaluating and monitoring search engine performance as a crucial aspect of building an effective tool.
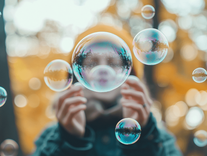
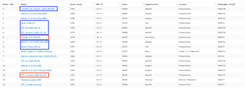
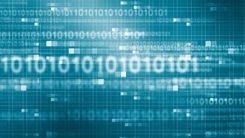
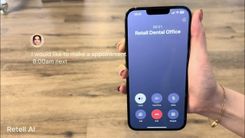
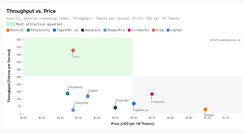