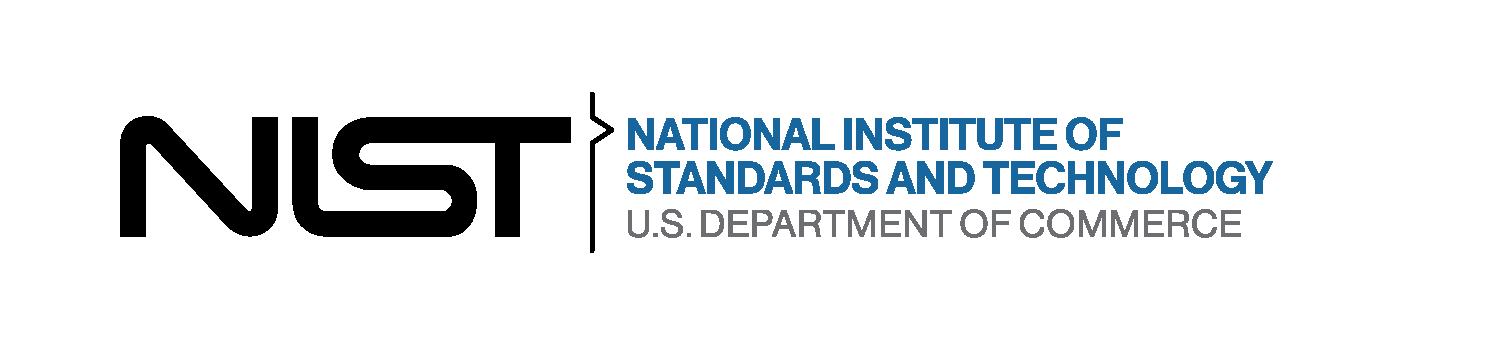
Adversarial Machine Learning: A Taxonomy and Terminology of Attacks and Mitigations
Date : 2024-01-12
Description
This summary was drafted with mixtral-8x7b-instruct-v0.1.Q5_K_M.gguf
This report provides a comprehensive report that develops a taxonomy for adversarial machine learning (AML). The taxonomy includes key types of ML methods, stages of attack, attacker goals and capabilities, and attacker knowledge. AML attacks are classified as evasion, poisoning, or privacy attacks, with corresponding mitigations discussed. The report highlights open challenges in the field, such as transferability of attacks between different models, systems, and datasets. Additionally, the authors provide a glossary that defines key terms associated with the security of AI systems, aiming to assist non-expert readers.
Read article here
Recently on :
Artificial Intelligence
Security | Surveillance | Privacy
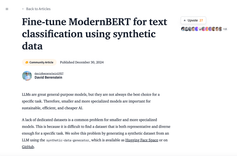
WEB - 2024-12-30
Fine-tune ModernBERT for text classification using synthetic data
David Berenstein explains how to finetune a ModernBERT model for text classification on a synthetic dataset generated from argi...
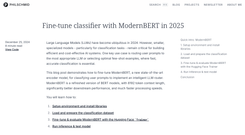
WEB - 2024-12-25
Fine-tune classifier with ModernBERT in 2025
In this blog post Philipp Schmid explains how to fine-tune ModernBERT, a refreshed version of BERT models, with 8192 token cont...
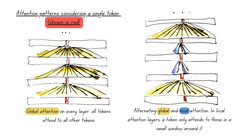
WEB - 2024-12-18
MordernBERT, finally a replacement for BERT
6 years after the release of BERT, answer.ai introduce ModernBERT, bringing modern model optimizations to encoder-only models a...
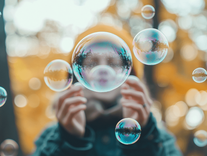
PITTI - 2024-09-19
A bubble in AI?
Bubble or true technological revolution? While the path forward isn't without obstacles, the value being created by AI extends ...
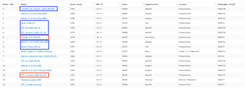
PITTI - 2024-09-08
Artificial Intelligence : what everyone can agree on
Artificial Intelligence is a divisive subject that sparks numerous debates about both its potential and its limitations. Howeve...