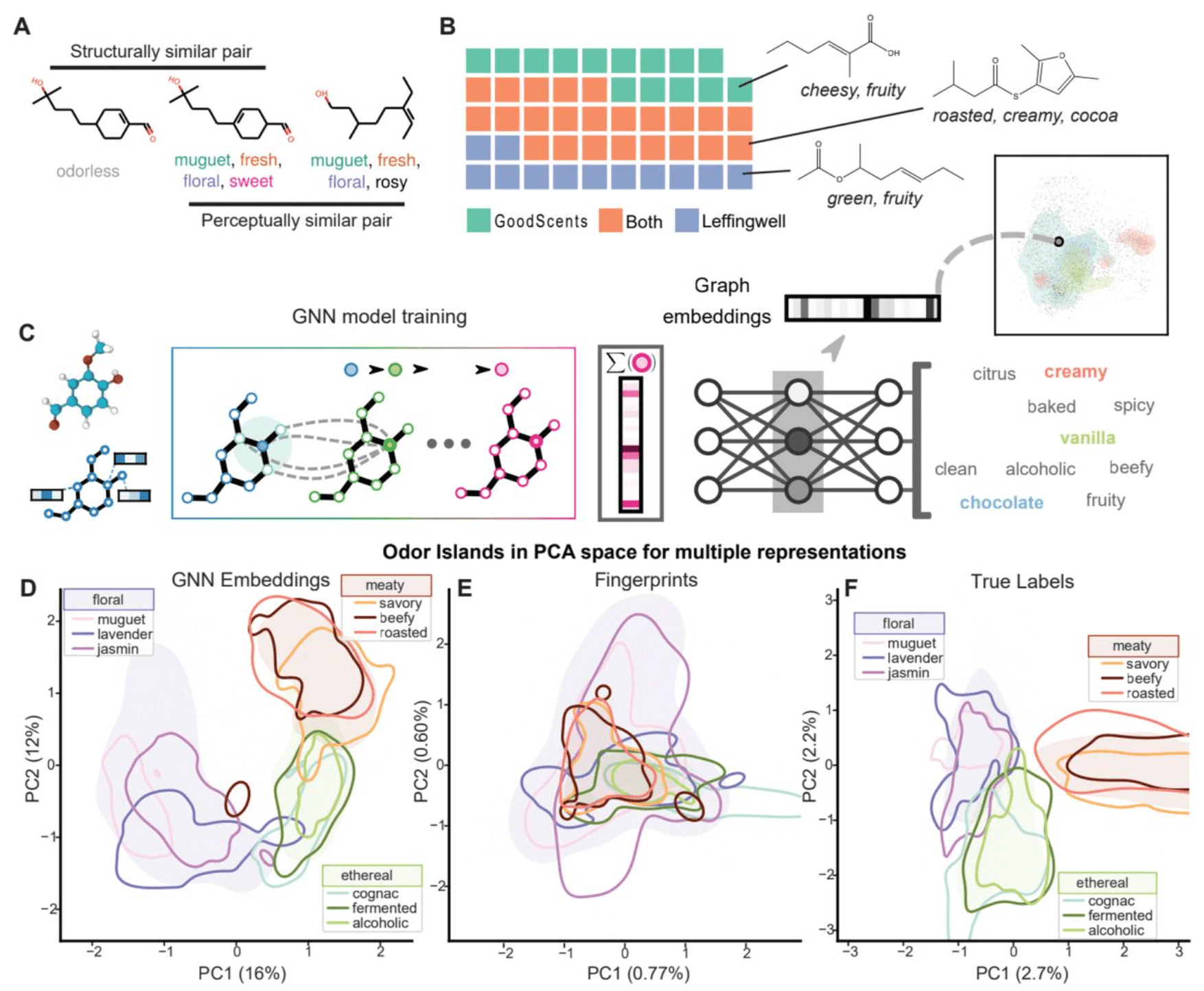
Abstract
Mapping molecular structure to odor perception is a key challenge in olfaction. Here, we use graph neural networks (GNN) to generate a Principal Odor Map (POM) that preserves perceptual relationships and enables odor quality prediction for novel odorants. The model is as reliable as a human in describing odor quality: on a prospective validation set of 400 novel odorants, the model-generated odor profile more closely matched the trained panel mean (n=15) than did the median panelist. Applying simple, interpretable, theoretically-rooted transformations, the POM outperformed chemoinformatic models on several other odor prediction tasks, indicating that the POM successfully encoded a generalized map of structure-odor relationships. This approach broadly enables odor prediction and paves the way toward digitizing odors.
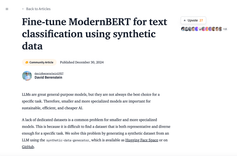
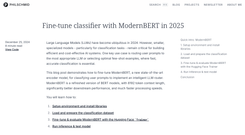
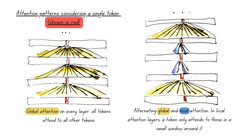
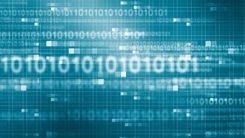
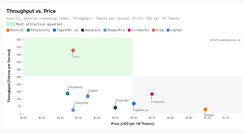