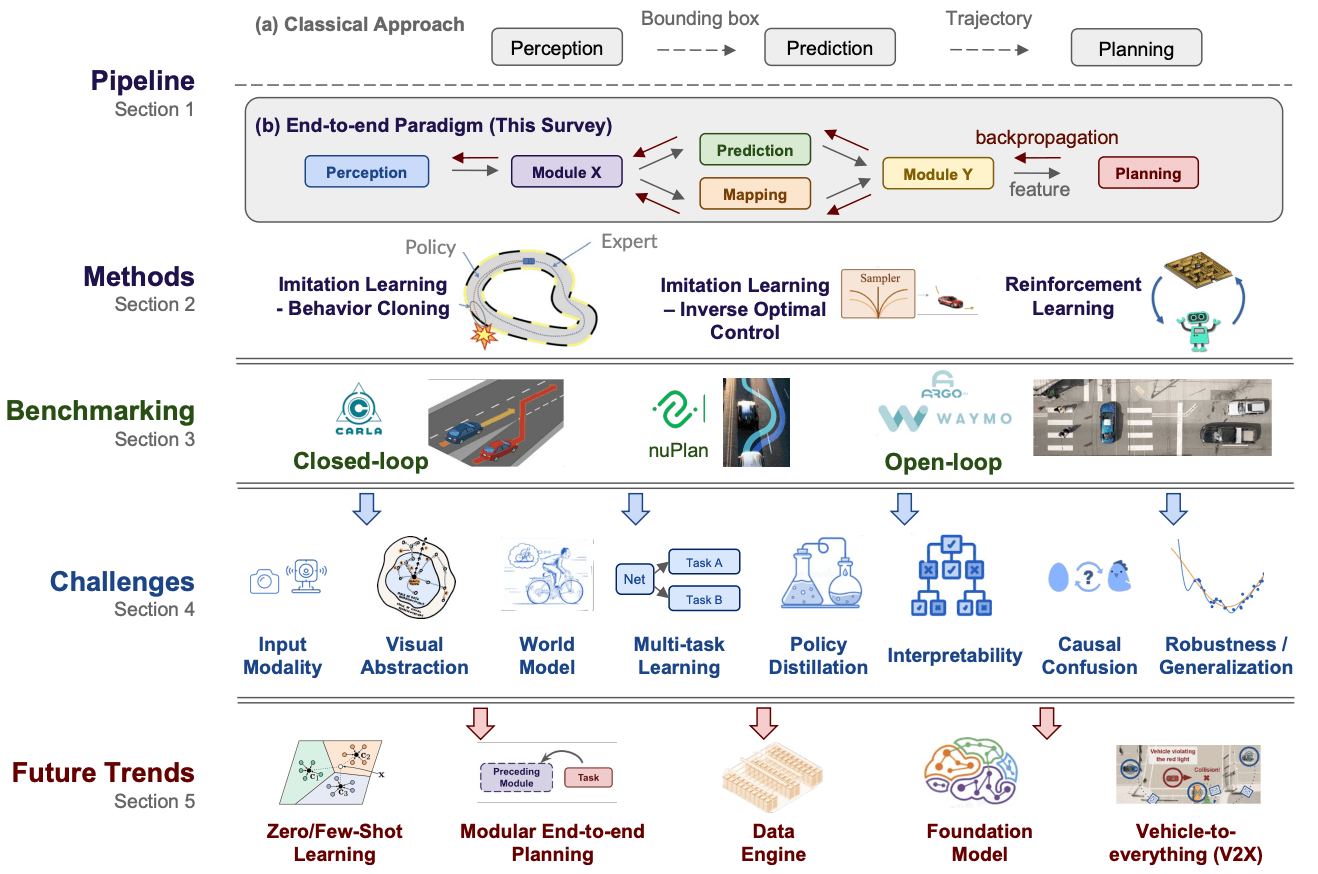
Abstract
The autonomous driving community has witnessed a rapid growth in approaches that embrace an end-to-end algorithm framework, utilizing raw sensor input to generate vehicle motion plans, instead of concentrating on individual tasks such as detection and motion prediction. End-to-end systems, in comparison to modular pipelines, benefit from joint feature optimization for perception and planning. This field has flourished due to the availability of large-scale datasets, closed-loop evaluation, and the increasing need for autonomous driving algorithms to perform effectively in challenging scenarios. In this survey, we provide a comprehensive analysis of more than 250 papers, covering the motivation, roadmap, methodology, challenges, and future trends in end-to-end autonomous driving. We delve into several critical challenges, including multi-modality, interpretability, causal confusion, robustness, and world models, amongst others. Additionally, we discuss current advancements in foundation models and visual pre-training, as well as how to incorporate these techniques within the end-to-end driving framework.
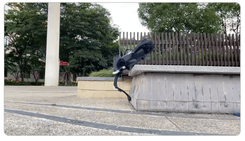
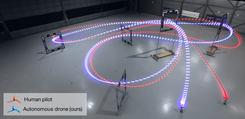
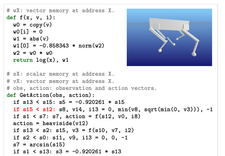